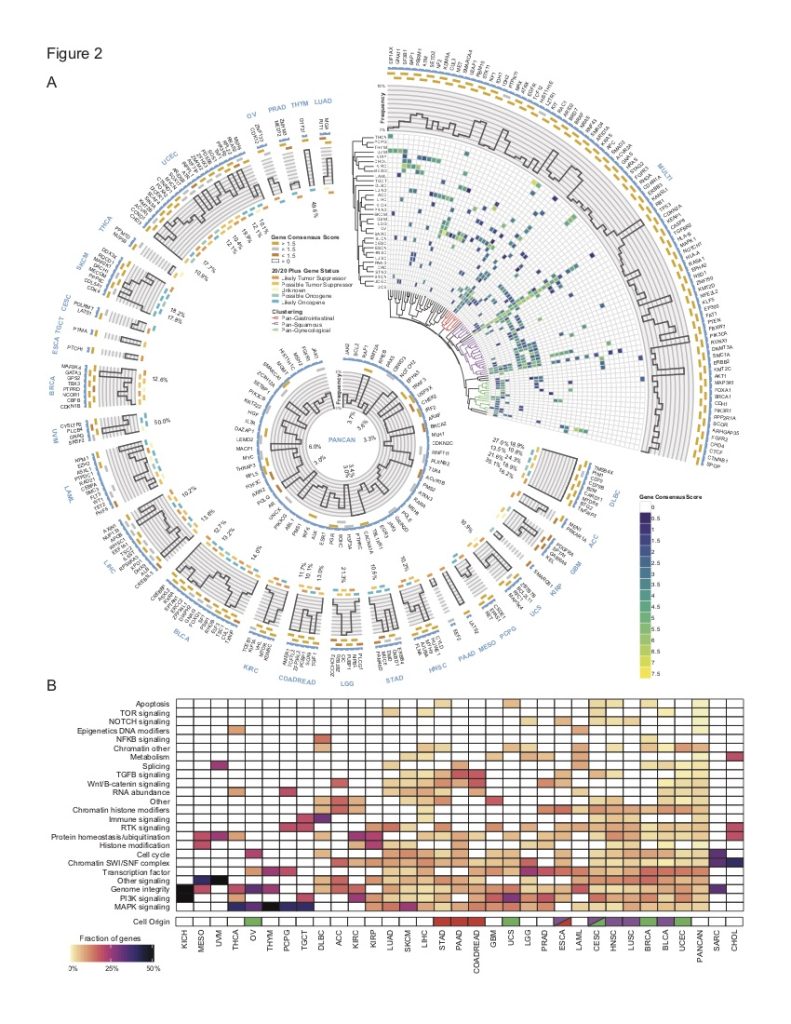
In one of the first efforts to use machine learning to interpret tumor sequencing data, we developed CHASM, a supervised learning algorithm for predicting driver missense mutations. The algorithm integrated information about evolutionary conservation, amino acid biochemistry, protein context, structural predictions, and annotations, and we demonstrated that it improved on the current state-of-the-art in missense mutation impact prediction. A redesigned version, CHASMplus, leveraged the statistical power of tens of thousands of tumor-derived mutations discovered after >10 years of large-scale tumor sequencing by the Cancer Genome Atlas (TCGA). In this work, we used semi-supervised machine learning and trained both a pan-cancer and 32 cancer-type specific classifiers. The cancer-type specific classifiers were the first to successfully discriminate driver mutations in different cancer types. We also developed and validated a supervised machine learning method to discover oncogene and tumor-suppressor driver genes, training on a large collection of publicly available mutation data. Many research groups have developed algorithms to identify cancer driver genes, but evaluation of these methods is difficult, due to lack of agreement on bona fide driver genes. We developed a new validation approach that could be applied in the absence of a “golden standard” by assessing prediction consistency, alignment with established and hypothesized cancer drivers, and adherence to expected p-value distributions.
In a culmination of the efforts of TCGA to identify driver genes and mutations, we worked with research groups throughout the U.S to develop and validate consensus methods to integrate 26 published computational tools and translate their predictions into a list of actionable mutations. A subset of the predictions was validated with an in vitro assay. The project identified a catalog of high-confidence driver genes (299) and driver mutations (>3400).