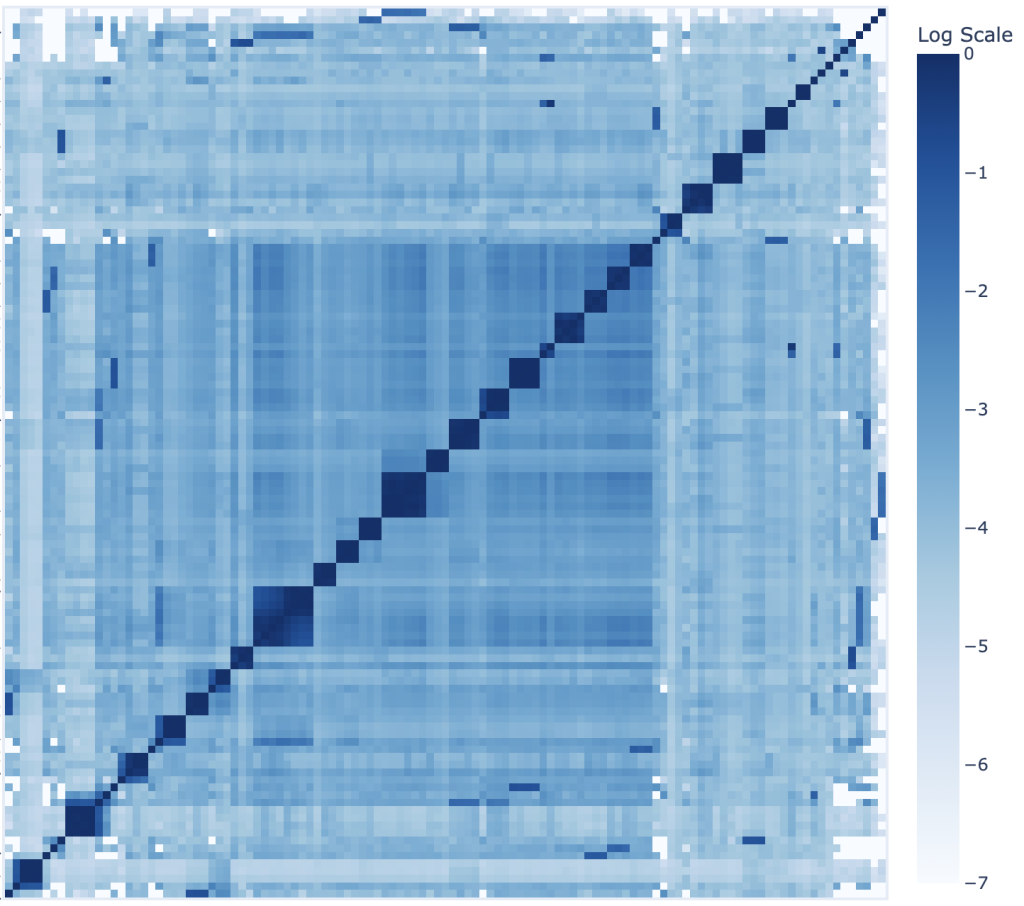
To understand how the diversity and composition of T cells affect the outcome of cancer treatment, we are developing tools for analysis of multi-omic data from bulk, single cell, and spatial T cell receptor (TCR) sequencing assays. TCRseq can identify variable regions on receptors on the surface of each T cell, enabling statistical analysis of T cell repertoire dynamics.
We are working with a large, collaborative team to create pipelines that ingest, analyze, and present TCR sequencing data with three main objectives: 1) to improve the reproducibility and transparency of data analysis, 2) ease-of-use for beginners, and 3) to better understand the biological mechanisms of cancer progression and remission. The pipelines are designed for bulk, single-cell, and spatial TCR sequencing data and are wrapped in NextFlow to be run in the cloud or in high-performance computing centers (HPCs).
We are also developing models of TCR antigen specificity and spatial evolution of the TCR repertoire. Current projects include: benchmarking of different approaches to TCR and antigen featurization: advanced BERT (Bidirectional Encoder Representations from Transformers) models trained with a Masked Language Model (MLM) objective, human-engineered features derived from prior immunological knowledge, and embeddings combined with Convolutional Neural Networks (CNNs); and building TCR evolution trees using spatial TCR sequencing, transcriptomics, and single-cell transcriptomics data.