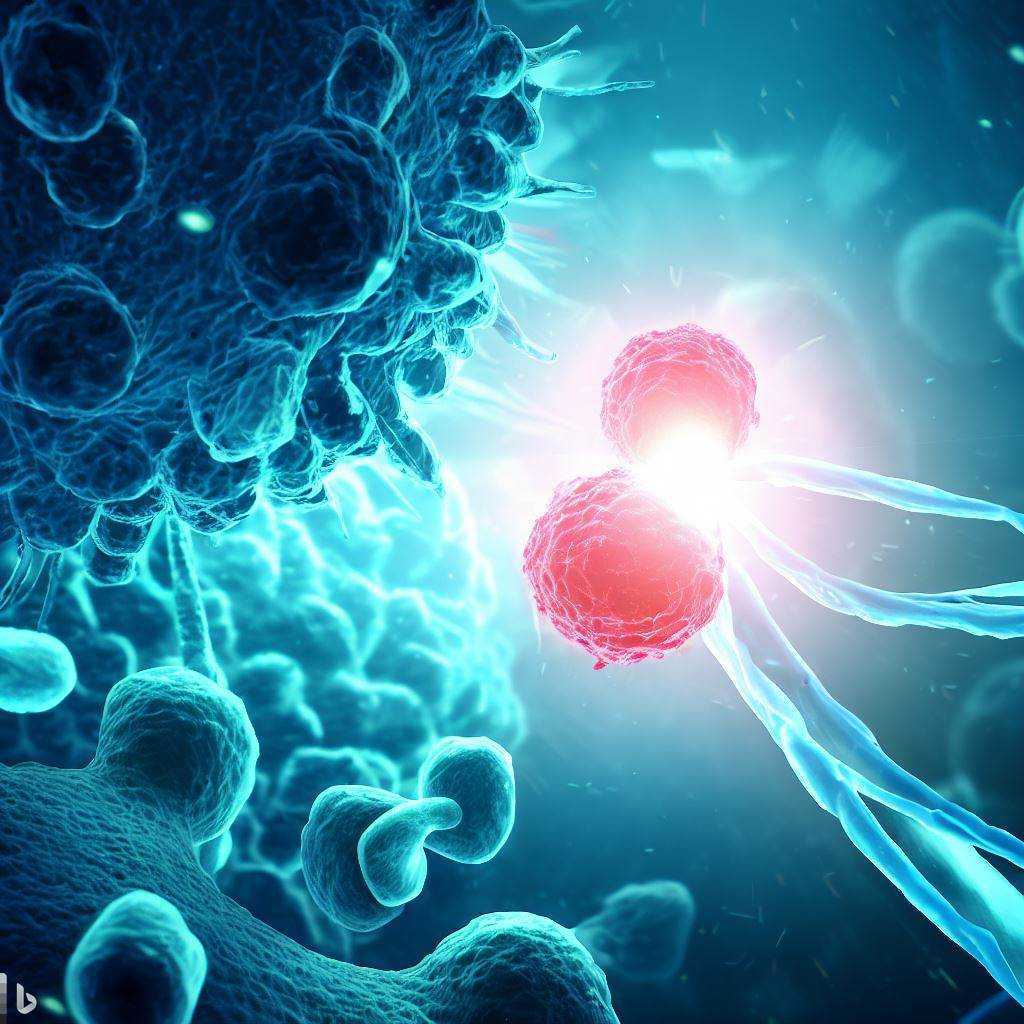
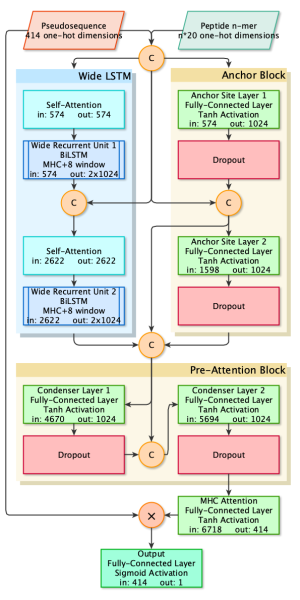
In the emerging field of precision cancer immunotherapies, understanding the basis for immune system recognition of tumor cells has become crucial. The primary signal with which T cells recognize tumor and professional antigen-presenting cells (APCs) is the binding of T cell receptors (TCRs) to neoantigen peptides presented by Major Histocompatibility Complex (MHC molecules) on the cell surface. While the intent of neoantigen prediction is to predict whether a peptide elicits T cell activation, most current predictors aim at the easier task of predicting which peptides will bind to or be presented by MHC molecules. We developed MHCnuggets, a deep-learning model capable of processing varying peptide lengths and predicting binding to both MHC I and II types. This model proved that a limited set of predicted MHC II neoantigens could discern between responders and non-responders to immune checkpoint therapy, outperforming traditional tumor mutation burden metrics in lung cancer studies. In our latest study, we introduced BigMHC, a deep neural network ensemble engineered to predict how T cells respond to specific antigens. We innovated a representation for MHC alleles by pinpointing key amino acid residues from an extensive cross-species sequence analysis. The networks were first trained on a very large peptide-MHC (pMHC) presentation dataset, identified via peptide elution and mass spectrometry. We then fine-tuned the networks through transfer learning with a curated dataset of pMHCs, experimentally validated to initiate T cell activation. This technique significantly outperformed existing methods in predicting T cell reactivity, as confirmed by ELIspot and MANAFEST assays.